AMD and Nvidia Part 2
AMD and Nvidia graphics cards are both popular choices for gamers, but when it comes to artificial intelligence (AI), the differences between the two become much more pronounced. While both companies have made efforts to optimize their cards for machine learning, Nvidia has been the clear leader in this field for years.
Nvidia’s GPUs are designed with a specific focus on AI, with features like Compute Unified Device Architecture (CUDA) cores and Tensor Cores that aid the performance of deep learning algorithms. CUDA cores are designed to work specifically with Nvidia’s parallel computing platform, which is only accessible to Nvidia graphics cards. This platform allows programmers to take advantage of the massive parallel processing power of Nvidia GPUs to run machine learning algorithms at high speeds.
In contrast, AMD’s cards use Compute Units (CUs) and Stream Processors (SPs) instead of CUDA cores. While AMD has made some efforts to catch up to Nvidia, such as with its Radeon Open Compute platform (ROCm) and the GPUFORT project, these initiatives are relatively recent and have not yet achieved the level of support enjoyed by Nvidia’s CUDA libraries.
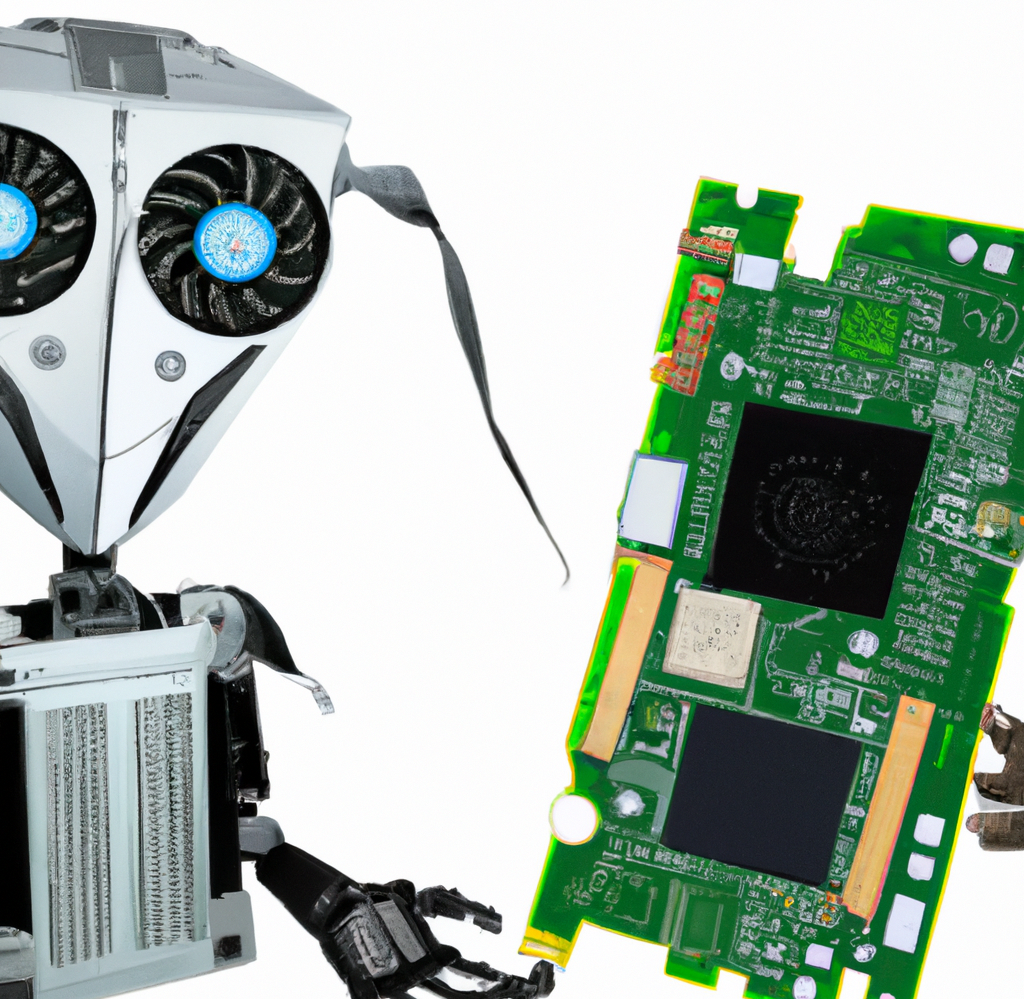
Nvidia’s dominance in the field of AI is not just due to the technical features of its GPUs, but also to the company’s long history of investment in the development of CUDA libraries. Most of the progress in AI in recent years has been made using Nvidia’s CUDA libraries, and the support for these libraries is much more widespread among the most popular deep learning frameworks.
While AMD’s efforts to catch up to Nvidia are commendable, the gap between the two companies only seems to grow wider each year. As long as Nvidia continues to invest in the development of CUDA libraries and optimize its GPUs for AI, it is likely to remain the dominant player in this field for the foreseeable future.